Written by Mina Yu, CRHESI Student Collective, Community Engaged Learning placement, Bachelor of Health Sciences, Western University
Artificial Intelligence (AI) has undoubtedly transformed healthcare. Its applications—from improving diagnostic accuracy and resource allocation to streamlining workflows—promise to revolutionize patient care. AI’s potential to reduce human error, anticipate healthcare needs, and optimize management of chronic diseases paints an optimistic picture of timely, equitable healthcare. However, beneath its transformative capabilities lies a critical challenge: algorithmic bias.
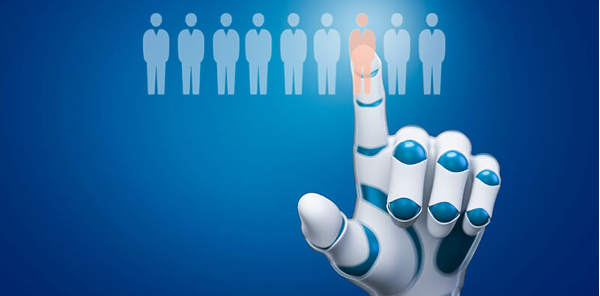
AI, lauded for its potential to minimize human biases and inconsistencies, often inherits the same inequities embedded in healthcare systems. When trained on homogeneous datasets or data that over-represent privileged groups, AI systems perpetuate existing biases, undermining efforts to address systemic disparities. For instance, a review by Celi et al. (2022) revealed that most clinical AI models are built on datasets from middle- and high-income countries, especially the U.S. and China. When the relationships within data sources used to train AI models differ from those in the populations where these models are deployed, the resulting outputs are often inaccurate. This mismatch restricts the effectiveness of AI systems, particularly in underserved and underrepresented communities, including but not limited to those in lower-income countries. Consequently, AI-driven interventions are systematically misaligned with the needs of these individuals and groups, resulting in inappropriate or inaccessible care. Rather than reducing disparities, this failure to provide suitable care exacerbates existing inequities.
One striking example involves an AI algorithm used by insurance companies to predict healthcare needs. A 2021 study (NIHCM Foundation) found that while Black patients were significantly sicker than their white counterparts, both groups were assigned similar risk scores. This bias stemmed from the algorithm’s reliance on past healthcare expenditures—a metric influenced by systemic inequities in access and treatment. Because Black patients, for structural and systemic reasons, typically receive less care despite generally experiencing worse health outcomes, their needs are inaccurately assessed. This perpetuates a harmful cycle of unmet healthcare needs, declining individual health, and widening disparities in health outcomes between populations.
For Canada, algorithmic bias intersects with the digital divide, disproportionately affecting rural, remote, and Indigenous Peoples, who are more likely that non-Indigenous people to live in remote Northern communities. This highlights gaps in socioeconomic and demographic factors, limiting these groups’ ability to benefit from AI advancements. Researchers like Anawati et al. (2024) emphasize that AI and machine learning literature is only beginning to address the absence of diversity in training data, and the real-world impacts on the health and well-being of groups already facing marginalization.
AI offers incredible promise, but its true potential lies in fostering equity, not reinforcing disparity. To bridge these gaps, recalibrating the fundamental assumptions of AI systems is essential. Incorporating diverse datasets from underserved groups and regions can help mitigate bias and extend AI’s benefits to marginalized populations. Ultimately, for AI to truly revolutionize healthcare, its development must be inclusive, addressing not just technical challenges but also the societal inequities that shape healthcare outcomes.
References
Anawati, A., Fleming, H., Mertz, M., Bertrand, J., Dumond, J., Myles, S., et al. (2024). Artificial intelligence and social accountability in the Canadian health care landscape: A rapid literature review. PLOS Digit Health, 3(9): e0000597. https://doi.org/10.1371/journal.pdig.0000597
Celi, L.A., Cellini, J., Charpignon, M.L., Dee, E.C., Dernoncourt, F., Eber, R., et al. (2022). Sources of bias in artificial intelligence that perpetuate healthcare disparities—A global review. PLOS Digital Health, 1(3): e0000022. https://doi.org/10.1371/journal.pdig.0000022
Igoe, K.J. (n.d.). Algorithmic Bias in Health Care Exacerbates Social Inequities — How to Prevent It. Artificial Intelligence and Technology. Retrieved from https://www.hsph.harvard.edu/ecpe/how-to-prevent-algorithmic-bias-in-health-care/
Johns Hopkins. (2023, May 02). How Health Care Algorithms and AI Can Help and Harm. Retrieved from https://publichealth.jhu.edu/2023/how-health-care-algorithms-and-ai-can-help-and-harm
Morales, K. (2022, January 5). Can Artificial Intelligence Help Increase Diversity in STEM? CSUF News. https://news.fullerton.edu/2022/01/can-artificial-intelligence-help-increase-diversity-in-stem/
NIHCM Foundation. (2021, Sept 30). Racial Bias in Health Care Artificial Intelligence. Artificial Intelligence. Retrieved from https://nihcm.org/publications/artificial-intelligences-racial-bias-in-health-care
Panch, T., Mattie, H., Atun, R. (2019). Artificial intelligence and algorithmic bias: implications for health systems. J Glob Health, 9(2):020318. https://doi.org/10.7189/jogh.09.020318